mirror of
https://github.com/meilisearch/MeiliSearch
synced 2024-06-03 03:18:03 +02:00
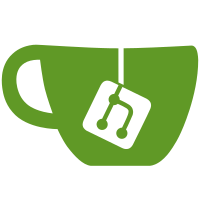
Instead of the user manually specifying the model dimensions it will now automatically get determined Just like with hf.rs the word "test" gets embedded to determine the dimensions of the output Add a dedicated error type for if the model doesn't exist (don't automatically pull it though) and set the fault of that error to be the user
308 lines
9.7 KiB
Rust
308 lines
9.7 KiB
Rust
// Copied from "openai.rs" with the sections I actually understand changed for Ollama.
|
|
// The common components of the Ollama and OpenAI interfaces might need to be extracted.
|
|
|
|
use std::fmt::Display;
|
|
|
|
use reqwest::StatusCode;
|
|
|
|
use super::error::{EmbedError, NewEmbedderError};
|
|
use super::openai::Retry;
|
|
use super::{DistributionShift, Embedding, Embeddings};
|
|
|
|
#[derive(Debug)]
|
|
pub struct Embedder {
|
|
headers: reqwest::header::HeaderMap,
|
|
options: EmbedderOptions,
|
|
}
|
|
|
|
#[derive(Debug, Clone, Hash, PartialEq, Eq, serde::Deserialize, serde::Serialize)]
|
|
pub struct EmbedderOptions {
|
|
pub embedding_model: EmbeddingModel,
|
|
}
|
|
|
|
#[derive(
|
|
Debug, Clone, Hash, PartialEq, Eq, serde::Serialize, serde::Deserialize, deserr::Deserr,
|
|
)]
|
|
#[deserr(deny_unknown_fields)]
|
|
pub struct EmbeddingModel {
|
|
name: String,
|
|
dimensions: usize,
|
|
}
|
|
|
|
#[derive(Debug, serde::Serialize)]
|
|
struct OllamaRequest<'a> {
|
|
model: &'a str,
|
|
prompt: &'a str,
|
|
}
|
|
|
|
#[derive(Debug, serde::Deserialize)]
|
|
struct OllamaResponse {
|
|
embedding: Embedding,
|
|
}
|
|
|
|
#[derive(Debug, serde::Deserialize)]
|
|
pub struct OllamaError {
|
|
error: String,
|
|
}
|
|
|
|
impl EmbeddingModel {
|
|
pub fn max_token(&self) -> usize {
|
|
// this might not be the same for all models
|
|
8192
|
|
}
|
|
|
|
pub fn default_dimensions(&self) -> usize {
|
|
// Dimensions for nomic-embed-text
|
|
768
|
|
}
|
|
|
|
pub fn name(&self) -> String {
|
|
self.name.clone()
|
|
}
|
|
|
|
pub fn from_name(name: &str) -> Self {
|
|
Self { name: name.to_string(), dimensions: 0 }
|
|
}
|
|
|
|
pub fn supports_overriding_dimensions(&self) -> bool {
|
|
false
|
|
}
|
|
}
|
|
|
|
impl Default for EmbeddingModel {
|
|
fn default() -> Self {
|
|
Self { name: "nomic-embed-text".to_string(), dimensions: 0 }
|
|
}
|
|
}
|
|
|
|
impl EmbedderOptions {
|
|
pub fn with_default_model() -> Self {
|
|
Self { embedding_model: Default::default() }
|
|
}
|
|
|
|
pub fn with_embedding_model(embedding_model: EmbeddingModel) -> Self {
|
|
Self { embedding_model }
|
|
}
|
|
}
|
|
|
|
impl Embedder {
|
|
pub fn new_client(&self) -> Result<reqwest::Client, EmbedError> {
|
|
reqwest::ClientBuilder::new()
|
|
.default_headers(self.headers.clone())
|
|
.build()
|
|
.map_err(EmbedError::openai_initialize_web_client)
|
|
}
|
|
|
|
pub fn new(options: EmbedderOptions) -> Result<Self, NewEmbedderError> {
|
|
let mut headers = reqwest::header::HeaderMap::new();
|
|
headers.insert(
|
|
reqwest::header::CONTENT_TYPE,
|
|
reqwest::header::HeaderValue::from_static("application/json"),
|
|
);
|
|
|
|
let mut embedder = Self { options, headers };
|
|
|
|
let rt = tokio::runtime::Builder::new_current_thread()
|
|
.enable_io()
|
|
.enable_time()
|
|
.build()
|
|
.map_err(EmbedError::openai_runtime_init)
|
|
.map_err(NewEmbedderError::ollama_could_not_determine_dimension)?;
|
|
|
|
// Get dimensions from Ollama
|
|
let request =
|
|
OllamaRequest { model: &embedder.options.embedding_model.name(), prompt: "test" };
|
|
// TODO: Refactor into shared error type
|
|
let client = embedder
|
|
.new_client()
|
|
.map_err(NewEmbedderError::ollama_could_not_determine_dimension)?;
|
|
|
|
rt.block_on(async move {
|
|
let response = client
|
|
.post(get_ollama_path())
|
|
.json(&request)
|
|
.send()
|
|
.await
|
|
.map_err(EmbedError::ollama_unexpected)
|
|
.map_err(NewEmbedderError::ollama_could_not_determine_dimension)?;
|
|
|
|
// Process error in case model not found
|
|
let response = Self::check_response(response).await.map_err(|_err| {
|
|
let e = EmbedError::ollama_model_not_found(OllamaError {
|
|
error: format!("model: {}", embedder.options.embedding_model.name()),
|
|
});
|
|
NewEmbedderError::ollama_could_not_determine_dimension(e)
|
|
})?;
|
|
|
|
let response: OllamaResponse = response
|
|
.json()
|
|
.await
|
|
.map_err(EmbedError::ollama_unexpected)
|
|
.map_err(NewEmbedderError::ollama_could_not_determine_dimension)?;
|
|
|
|
let embedding = Embeddings::from_single_embedding(response.embedding);
|
|
|
|
embedder.options.embedding_model.dimensions = embedding.dimension();
|
|
|
|
tracing::info!(
|
|
"ollama model {} with dimensionality {} added",
|
|
embedder.options.embedding_model.name(),
|
|
embedding.dimension()
|
|
);
|
|
|
|
Ok(embedder)
|
|
})
|
|
}
|
|
|
|
async fn check_response(response: reqwest::Response) -> Result<reqwest::Response, Retry> {
|
|
if !response.status().is_success() {
|
|
// Not the same number of possible error cases covered as with OpenAI.
|
|
match response.status() {
|
|
StatusCode::TOO_MANY_REQUESTS => {
|
|
let error_response: OllamaError = response
|
|
.json()
|
|
.await
|
|
.map_err(EmbedError::ollama_unexpected)
|
|
.map_err(Retry::retry_later)?;
|
|
|
|
return Err(Retry::rate_limited(EmbedError::ollama_too_many_requests(
|
|
OllamaError { error: error_response.error },
|
|
)));
|
|
}
|
|
StatusCode::SERVICE_UNAVAILABLE => {
|
|
let error_response: OllamaError = response
|
|
.json()
|
|
.await
|
|
.map_err(EmbedError::ollama_unexpected)
|
|
.map_err(Retry::retry_later)?;
|
|
return Err(Retry::retry_later(EmbedError::ollama_internal_server_error(
|
|
OllamaError { error: error_response.error },
|
|
)));
|
|
}
|
|
StatusCode::NOT_FOUND => {
|
|
let error_response: OllamaError = response
|
|
.json()
|
|
.await
|
|
.map_err(EmbedError::ollama_unexpected)
|
|
.map_err(Retry::give_up)?;
|
|
|
|
return Err(Retry::give_up(EmbedError::ollama_model_not_found(OllamaError {
|
|
error: error_response.error,
|
|
})));
|
|
}
|
|
code => {
|
|
return Err(Retry::give_up(EmbedError::ollama_unhandled_status_code(
|
|
code.as_u16(),
|
|
)));
|
|
}
|
|
}
|
|
}
|
|
Ok(response)
|
|
}
|
|
|
|
pub async fn embed(
|
|
&self,
|
|
texts: Vec<String>,
|
|
client: &reqwest::Client,
|
|
) -> Result<Vec<Embeddings<f32>>, EmbedError> {
|
|
// Ollama only embedds one document at a time.
|
|
let mut results = Vec::with_capacity(texts.len());
|
|
|
|
// The retry loop is inside the texts loop, might have to switch that around
|
|
for text in texts {
|
|
// Retries copied from openai.rs
|
|
for attempt in 0..7 {
|
|
let retry_duration = match self.try_embed(&text, client).await {
|
|
Ok(result) => {
|
|
results.push(result);
|
|
break;
|
|
}
|
|
Err(retry) => {
|
|
tracing::warn!("Failed: {}", retry.error);
|
|
retry.into_duration(attempt)
|
|
}
|
|
}?;
|
|
tracing::warn!(
|
|
"Attempt #{}, retrying after {}ms.",
|
|
attempt,
|
|
retry_duration.as_millis()
|
|
);
|
|
tokio::time::sleep(retry_duration).await;
|
|
}
|
|
}
|
|
|
|
Ok(results)
|
|
}
|
|
|
|
async fn try_embed(
|
|
&self,
|
|
text: &str,
|
|
client: &reqwest::Client,
|
|
) -> Result<Embeddings<f32>, Retry> {
|
|
let request = OllamaRequest { model: &self.options.embedding_model.name(), prompt: text };
|
|
let response = client
|
|
.post(get_ollama_path())
|
|
.json(&request)
|
|
.send()
|
|
.await
|
|
.map_err(EmbedError::openai_network)
|
|
.map_err(Retry::retry_later)?;
|
|
|
|
let response = Self::check_response(response).await?;
|
|
|
|
let response: OllamaResponse = response
|
|
.json()
|
|
.await
|
|
.map_err(EmbedError::openai_unexpected)
|
|
.map_err(Retry::retry_later)?;
|
|
|
|
tracing::trace!("response: {:?}", response.embedding);
|
|
|
|
let embedding = Embeddings::from_single_embedding(response.embedding);
|
|
Ok(embedding)
|
|
}
|
|
|
|
pub fn embed_chunks(
|
|
&self,
|
|
text_chunks: Vec<Vec<String>>,
|
|
) -> Result<Vec<Vec<Embeddings<f32>>>, EmbedError> {
|
|
let rt = tokio::runtime::Builder::new_current_thread()
|
|
.enable_io()
|
|
.enable_time()
|
|
.build()
|
|
.map_err(EmbedError::openai_runtime_init)?;
|
|
let client = self.new_client()?;
|
|
rt.block_on(futures::future::try_join_all(
|
|
text_chunks.into_iter().map(|prompts| self.embed(prompts, &client)),
|
|
))
|
|
}
|
|
|
|
// Defaults copied from openai.rs
|
|
pub fn chunk_count_hint(&self) -> usize {
|
|
10
|
|
}
|
|
|
|
pub fn prompt_count_in_chunk_hint(&self) -> usize {
|
|
10
|
|
}
|
|
|
|
pub fn dimensions(&self) -> usize {
|
|
self.options.embedding_model.dimensions
|
|
}
|
|
|
|
pub fn distribution(&self) -> Option<DistributionShift> {
|
|
None
|
|
}
|
|
}
|
|
|
|
impl Display for OllamaError {
|
|
fn fmt(&self, f: &mut std::fmt::Formatter<'_>) -> std::fmt::Result {
|
|
write!(f, "{}", self.error)
|
|
}
|
|
}
|
|
|
|
fn get_ollama_path() -> String {
|
|
// Important: Hostname not enough, has to be entire path to embeddings endpoint
|
|
std::env::var("MEILI_OLLAMA_URL").unwrap_or("http://localhost:11434/api/embeddings".to_string())
|
|
}
|